In today’s data-driven world, leveraging the power of artificial intelligence (AI) is essential for extracting meaningful insights from vast datasets. Businesses and researchers alike are increasingly turning to AI tools for data analysis to streamline their workflows and make data-driven decisions. In this article, we will explore the best AI tools for data analysis, providing a detailed overview of each tool’s capabilities, strengths, and use cases.
Introduction
As organizations accumulate ever-growing volumes of data, the need for effective data analysis tools becomes paramount. Traditional methods often fall short in handling the complexity and scale of modern datasets. This is where AI analytics tools come into play, offering sophisticated algorithms and machine learning techniques to uncover patterns, trends, and actionable insights. Whether you’re a data scientist, business analyst, or researcher, the following AI tools can revolutionize your data analysis process.
1. Tableau
Tableau is renowned for its powerful data visualization capabilities, allowing users to create interactive and shareable dashboards, making complex data more accessible and understandable. It connects to various data sources, such as databases, cloud services, and spreadsheets, with an easy-to-use drag-and-drop interface that suits both beginners and experienced analysts.
Key Features
- Interactive Dashboards: Create shareable and interactive dashboards.
- Data Connectivity: Connects to various data sources such as databases, cloud services, and spreadsheets.
- User-Friendly Interface: Drag-and-drop functionality for ease of use.
Strengths
- Helps in making complex data more accessible and understandable.
- Suitable for both beginners and experienced analysts.
Pros
- Excellent data visualization capabilities.
- Supports a wide range of data sources.
- User-friendly interface.
Cons
- Can be expensive for large teams.
- Requires some training for advanced features.
Requirements
- Requires a modern web browser for cloud version or a compatible operating system for the desktop version.
- Sufficient RAM and CPU for handling large datasets.
Use Cases
- Business intelligence and reporting.
- Real-time data analysis.
- Data-driven decision-making.
Related Post : Best AI Tools For Content Writing
2. Polymer
Polymer offers a unique approach to data analysis by providing an intuitive, no-code platform, allowing users to integrate, analyze, and visualize data effortlessly. It is particularly useful for small to medium-sized enterprises looking to harness the power of AI without the need for extensive technical expertise.
Key Features
- No-Code Platform: Allows users to analyze and visualize data without coding.
- AI-Driven Insights: Provides automated insights to facilitate decision-making.
- Integration Capabilities: Seamlessly integrates with various data sources.
Strengths
- Intuitive interface, ideal for non-technical users.
- Quick setup and deployment.
- Suitable for small to medium-sized enterprises.
Pros
- No coding required.
- Fast implementation.
- Cost-effective for smaller teams.
Cons
- Limited customization options compared to some other tools.
- May not be suitable for very large datasets.
Requirements
- A web browser and internet connection.
- Basic understanding of data analysis concepts.
Use Cases
- Market research and analysis.
- Sales and marketing analytics.
- Operational efficiency improvements.
3. Power BI
Microsoft’s Power BI is a comprehensive business analytics tool that combines AI capabilities with robust data visualization features. It supports a wide range of data sources and offers advanced analytics through machine learning models. Power BI’s integration with other Microsoft products makes it an excellent choice for organizations already using the Microsoft ecosystem.
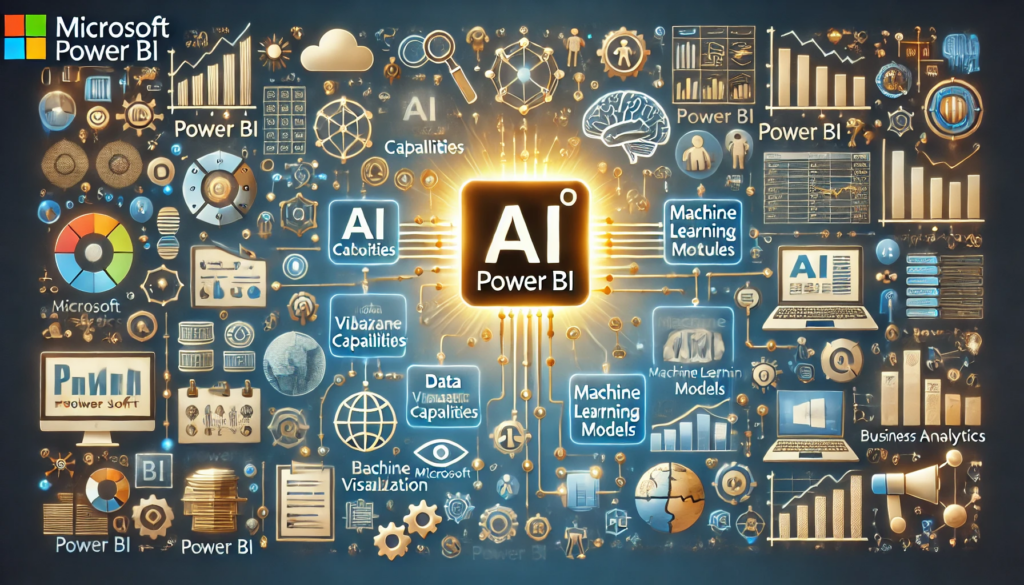
Key Features
- Comprehensive Analytics: Combines AI with robust data visualization.
- Wide Data Source Support: Connects to multiple data sources including databases and cloud services.
- Integration: Works seamlessly with other Microsoft products.
Strengths
- Powerful analytics and visualization tools.
- Strong integration with the Microsoft ecosystem.
- Scalable for businesses of all sizes.
Pros
- Extensive data source support.
- Seamless integration with Microsoft Office Suite.
- User-friendly for those familiar with Microsoft products.
Cons
- Can be complex for beginners.
- Subscription-based pricing can add up.
Requirements
- Requires Microsoft account for full functionality.
- Compatible with Windows OS, and available on Mac and mobile devices with limited features.
Use Cases
- Enterprise reporting and analytics.
- Financial analysis.
- Performance tracking and monitoring.
4. Akkio Inc.
Akkio Inc. specializes in no-code AI solutions for data analysis, enabling users to build and deploy machine learning models with ease. Its automated data preprocessing and model selection features make it accessible to users with minimal AI expertise. This AI data analysis tool is ideal for businesses seeking to implement AI-driven insights quickly.
Key Features
- Automated Machine Learning: No-code platform for building and deploying ML models.
- Data Preprocessing: Automates data cleaning and preparation.
- Model Selection: Helps in choosing the best model for your data.
Strengths
- Easy to use, even for those with minimal AI expertise.
- Quick implementation of AI-driven insights.
- Reduces the time and effort needed for model building.
Pros
- No coding required.
- Fast and efficient model building.
- User-friendly interface.
Cons
- Limited customization for advanced users.
- May not handle very large datasets as efficiently.
Requirements
- Internet connection and web browser.
- Basic understanding of machine learning concepts.
Use Cases
- Predictive analytics for business operations.
- Customer behavior analysis.
- Marketing campaign optimization.
5. MonkeyLearn
MonkeyLearn is an AI tool for data analysis that focuses on text analytics. It provides tools for sentiment analysis, topic classification, and entity extraction, among other text-related tasks. MonkeyLearn’s user-friendly interface allows users to create custom text analysis models without coding. It’s particularly useful for analyzing customer feedback, social media data, and other textual information.
Key Features
- Text Analysis: Offers tools for sentiment analysis, topic classification, and entity extraction.
- Customizable Models: Allows users to create and train custom text analysis models.
- User-Friendly Interface: No coding required for model creation.
Strengths
- Specializes in text analytics.
- Easy to implement and use.
- Versatile for various text data sources.
Pros
- Powerful text analysis capabilities.
- Customizable models.
- No coding required.
Cons
- Limited to text data analysis.
- Can become costly with extensive use.
Requirements
- Web browser and internet connection.
- Basic knowledge of text analysis concepts.
Use Cases
- Analyzing customer feedback and reviews.
- Social media monitoring and sentiment analysis.
- Automated text classification and tagging.
6. KNIME
KNIME (Konstanz Information Miner) is an open-source platform for data analytics and machine learning. It offers a visual workflow interface, enabling users to create data pipelines and machine learning models through drag-and-drop functionalities. KNIME’s extensive library of nodes and integrations makes it a versatile tool for a wide range of data analysis tasks.
Key Features
- Visual Workflow Interface: Drag-and-drop functionality for creating data pipelines.
- Open-Source Platform: Extensive library of nodes for various data tasks.
- Integration: Connects with numerous data sources and ML libraries.
Strengths
- Highly customizable and versatile.
- Strong community support and resources.
- Suitable for a wide range of data analysis tasks.
Pros
- Free and open-source.
- Extensive integration options.
- Strong community support.
Cons
- Can be complex for beginners.
- Requires more technical knowledge for advanced features.
Requirements
- Compatible operating system (Windows, Mac, Linux).
- Java runtime environment.
Use Cases
- Data preprocessing and transformation.
- Machine learning model development.
- Research and academic projects.
7. IBM Watson Analytics
IBM Watson Analytics leverages the power of IBM’s AI capabilities to provide advanced data analysis and visualization. It offers automated data preparation, natural language processing, and predictive analytics. Watson Analytics simplifies complex data tasks, making it easier for users to uncover insights and trends without deep technical knowledge.
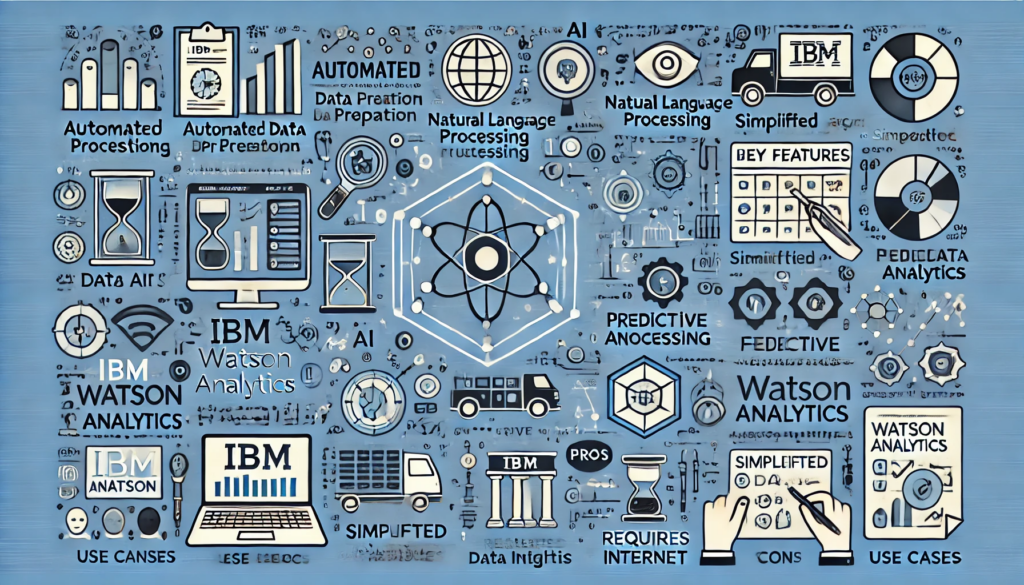
Key Features
- Automated Data Preparation: Simplifies data cleaning and preparation.
- Natural Language Processing: Enables users to interact with data using natural language queries.
- Predictive Analytics: Provides advanced forecasting and trend analysis.
Strengths
- Leverages IBM’s advanced AI capabilities.
- User-friendly, even for non-technical users.
- Comprehensive analytics features.
Pros
- Advanced AI capabilities.
- Simplifies data preparation and analysis.
- User-friendly interface.
Cons
- Can be expensive.
- Requires internet connection.
Requirements
- Internet connection and web browser.
- IBM account for full functionality.
Use Cases
- Business intelligence and strategy.
- Customer insights and trend analysis.
- Operational efficiency and optimization.
8. RapidMiner
RapidMiner is a data science platform that supports the entire analytics lifecycle, from data preparation to model deployment. It offers a visual workflow designer and integrates with numerous data sources and machine learning libraries. RapidMiner’s robust feature set makes it suitable for both beginners and experienced data scientists.
Key Features
- Visual Workflow Designer: Easy-to-use interface for building data workflows.
- End-to-End Platform: Supports the entire analytics lifecycle.
- Integration: Compatible with numerous data sources and ML libraries.
Strengths
- Robust feature set for comprehensive data analysis.
- Suitable for both novice and experienced data scientists.
- Strong focus on collaboration and sharing.
Pros
- Comprehensive toolset for data science.
- Strong integration capabilities.
- User-friendly interface.
Cons
- Can be resource-intensive.
- May require training for advanced features.
Requirements
- Compatible operating system (Windows, Mac, Linux).
- Sufficient RAM and CPU for large datasets.
Use Cases
- Predictive maintenance in manufacturing.
- Financial risk modeling.
- Customer segmentation and analysis.
9. Sisense
Sisense is a business intelligence tool that incorporates AI-driven analytics to help users uncover insights from their data. It offers powerful data integration, visualization, and dashboarding capabilities. Sisense’s AI features, such as predictive analytics and natural language query, enhance its usability for data-driven decision-making.
Key Features
- AI-Driven Analytics: Integrates AI to enhance data insights.
- Powerful Visualization: Offers advanced data visualization capabilities.
- Real-Time Data Processing: Handles real-time data for up-to-date insights.
Strengths
- Scalable for large datasets.
- Intuitive interface for creating interactive dashboards.
- Strong data integration capabilities.
Pros
- Excellent real-time data processing.
- User-friendly dashboard creation.
- Strong integration capabilities.
Cons
- Can be expensive for larger organizations.
- May require training for advanced features.
Requirements
- Internet connection and web browser.
- Adequate hardware for processing large datasets.
Use Cases
- Real-time business intelligence.
- Operational reporting.
- Sales and marketing analytics.
10. DataRobot, Inc.
DataRobot, Inc. is an AI-driven platform designed to automate the end-to-end process of building, deploying, and maintaining machine learning models. It provides tools for data preprocessing, feature engineering, and model evaluation. DataRobot’s automated machine learning (AutoML) capabilities make it a valuable asset for organizations looking to accelerate their AI initiatives.
Key Features
- Automated Machine Learning: Automates the building and deployment of ML models.
- Feature Engineering: Provides tools for automated feature engineering.
- Model Evaluation: Helps in evaluating and selecting the best models.
Strengths
- Accelerates the AI deployment process.
- User-friendly for non-experts.
- Comprehensive suite for end-to-end machine learning.
Pros
- Fast and efficient ML model development.
- User-friendly interface.
- Strong feature engineering capabilities.
Cons
- Can be costly for extensive use.
- Limited customization for advanced users.
Requirements
- Internet connection and web browser.
- Basic understanding of machine learning concepts.
Use Cases
- Fraud detection in finance.
- Predictive maintenance in industrial applications.
- Customer churn prediction.
11. Qlik
Qlik offers a powerful AI data analysis tool that combines data visualization with advanced analytics. Its associative engine allows users to explore data relationships intuitively. Qlik’s AI features, such as predictive analytics and cognitive engine, enhance its ability to provide actionable insights from complex datasets.
Key Features
- Associative Engine: Enables intuitive exploration of data relationships.
- Advanced Analytics: Offers predictive analytics and cognitive engine capabilities.
- Data Visualization: Provides powerful tools for data visualization.
Strengths
- Facilitates in-depth data exploration.
- Strong AI capabilities for enhanced analytics.
- Suitable for large-scale data analysis.
Pros
- Intuitive data exploration.
- Advanced analytics features.
- Strong data visualization tools.
Cons
- Can be complex for beginners.
- May require significant investment.
Requirements
- Compatible operating system and internet connection.
- Basic understanding of data analysis concepts.
Use Cases
- Business intelligence and reporting.
- Customer insights and behavior analysis.
- Financial performance analysis.
12. AnswerRocket
AnswerRocket uses natural language processing and machine learning to provide AI-driven analytics. It allows users to ask questions in natural language and receive insights in real-time. AnswerRocket’s intuitive interface and advanced analytics capabilities make it a valuable tool for business users and analysts.
Key Features
- Natural Language Processing: Allows users to query data using natural language.
- Real-Time Insights: Provides real-time analytics and insights.
- User-Friendly Interface: Easy to use, even for non-technical users.
Strengths
- Quick access to insights through natural language queries.
- User-friendly for business users and analysts.
- Reduces the need for specialized data expertise.
Pros
- Easy to use.
- Real-time insights.
- Natural language processing capabilities.
Cons
- Limited customization for complex queries.
- Can be expensive for larger teams.
Requirements
- Internet connection and web browser.
- Basic understanding of business analytics.
Use Cases
- Sales and marketing analytics.
- Customer service optimization.
- Business performance tracking.
13. Google Cloud Platform
Google Cloud Platform (GCP) offers a suite of AI tools for data analysis, including BigQuery, AutoML, and AI Platform. These tools enable users to perform large-scale data analysis, build and deploy machine learning models, and leverage Google’s powerful infrastructure. GCP’s integration with other Google services makes it a flexible and scalable solution for data analysis.
Key Features
- BigQuery: Scalable data warehouse with real-time analytics.
- AutoML: Automated machine learning tools for model development.
- AI Platform: Comprehensive suite for AI model building and deployment.
Strengths
- Highly scalable and flexible infrastructure.
- Seamless integration with other Google services.
- Extensive toolset for data analysis and machine learning.
Pros
- Powerful and scalable.
- Wide range of AI tools.
- Strong integration with Google services.
Cons
- Can be complex for beginners.
- Requires technical expertise for advanced features.
Requirements
- Google account for full functionality.
- Internet connection and web browser.
Use Cases
- Large-scale data processing and analysis.
- Machine learning model development and deployment.
- Real-time data insights and reporting.
14. PyTorch
PyTorch is an open-source machine learning library developed by Facebook’s AI Research lab. It is widely used for deep learning applications, including natural language processing, computer vision, and reinforcement learning. PyTorch’s dynamic computational graph and extensive library support make it a popular choice for researchers and practitioners.
Key Features
- Dynamic Computational Graph: Supports dynamic and flexible model building.
- Extensive Library Support: Access to numerous pre-built models and libraries.
- Community and Documentation: Strong community support and extensive documentation.
Strengths
- Popular choice for research and development in deep learning.
- Highly flexible and adaptable.
- Extensive resources and community support.
Pros
- Strong community support.
- Flexible and dynamic.
- Wide range of applications.
Cons
- Steeper learning curve for beginners.
- Requires programming knowledge.
Requirements
- Python programming environment.
- Compatible operating system (Windows, Mac, Linux).
Use Cases
- Academic research in AI and machine learning.
- Development of deep learning applications.
- Natural language processing and computer vision projects.
Also Read: 10 Best AI Tools For Making Presentations
15. ChatGPT
ChatGPT, developed by OpenAI, is an AI tool for data analysis that excels in natural language understanding and generation. It can assist with data exploration, report generation, and answering data-related queries. ChatGPT’s conversational abilities make it a versatile tool for enhancing data analysis workflows.
Key Features
- Natural Language Understanding: Processes and understands human language queries.
- Conversational AI: Engages in interactive dialogues for data analysis.
- Versatile Integration: Can be integrated into various data analysis workflows.
Strengths
- Enhances data exploration through natural language interaction.
- Versatile use cases in various domains.
- User-friendly for non-technical users.
Pros
- Natural language interaction.
- Versatile and flexible.
- Easy to use.
Cons
- Limited to conversational applications.
- Requires internet connection.
Requirements
- Internet connection and web browser.
- Basic understanding of data analysis concepts.
Use Cases
- Data exploration and query answering.
- Automated report generation.
- Enhancing customer support with AI-driven insights.
16. Databricks
Databricks is a unified analytics platform that combines data engineering, machine learning, and business analytics. Built on Apache Spark, it offers robust data processing capabilities and collaborative features. Databricks’ integration with various data sources and its scalable infrastructure make it ideal for large-scale data analysis projects.
Key Features
- Unified Analytics Platform: Combines data engineering, ML, and analytics.
- Apache Spark Integration: Leverages the power of Spark for large-scale data processing.
- Collaborative Environment: Supports collaborative workflows and sharing.
Strengths
- Powerful and scalable infrastructure for data analysis.
- Collaborative features for team-based projects.
- Integration with various data sources and tools.
Pros
- Scalable and flexible.
- Strong collaboration features.
- Extensive integration options.
Cons
- Can be expensive.
- Requires technical expertise.
Requirements
- Internet connection and web browser.
- Compatible operating system (Windows, Mac, Linux).
Use Cases
- Large-scale data processing and analytics.
- Machine learning model development and deployment.
- Collaborative data science projects.
17. Jupyter AI
Jupyter AI extends the capabilities of Jupyter notebooks by incorporating AI-driven features. It allows users to perform interactive data analysis, build machine learning models, and visualize results within a single environment. Jupyter AI’s flexibility and integration with popular libraries make it a valuable tool for data scientists and researchers.
Key Features
- Interactive Notebooks: Supports interactive data analysis and visualization.
- AI-Driven Features: Enhances Jupyter notebooks with AI capabilities.
- Integration with Libraries: Compatible with popular data science libraries.
Strengths
- Flexible and interactive environment for data science.
- Enhances productivity with AI-driven features.
- Strong integration with existing tools and libraries.
Pros
- Interactive and flexible.
- Extensive library support.
- User-friendly for data scientists.
Cons
- Requires programming knowledge.
- Can be resource-intensive.
Requirements
- Python programming environment.
- Compatible operating system (Windows, Mac, Linux).
Use Cases
- Interactive data analysis and visualization.
- Development of machine learning models.
- Educational and research projects.
18. Luzmo
Luzmo is an AI-powered analytics platform that focuses on providing real-time insights. It offers data integration, visualization, and advanced analytics features. Luzmo’s AI-driven recommendations and predictive analytics capabilities help users make informed decisions quickly.
Key Features
- Real-Time Insights: Provides up-to-date analytics and insights.
- Data Integration: Seamlessly integrates with various data sources.
- Advanced Visualization: Offers powerful tools for data visualization.
Strengths
- Quick access to real-time data insights.
- Intuitive interface for creating interactive dashboards.
- Strong data integration capabilities.
Pros
- Real-time analytics.
- User-friendly interface.
- Strong data integration.
Cons
- Can be costly for larger datasets.
- Requires training for advanced features.
Requirements
- Internet connection and web browser.
- Basic understanding of data analysis.
Use Cases
- Real-time business intelligence and reporting.
- Operational monitoring and analysis.
- Sales and marketing analytics.
19. Talend
Talend is a data integration platform that incorporates AI and machine learning for data analysis. It provides tools for data cleansing, transformation, and visualization. Talend’s robust integration capabilities and AI-driven features make it a comprehensive solution for data analysis and management.
Key Features
- Data Integration: Integrates with various data sources for seamless data flow.
- AI-Driven Data Cleansing: Automates data cleaning and preparation.
- Data Transformation: Provides tools for transforming and enriching data.
Strengths
- Comprehensive data integration and management.
- AI-driven features for enhanced data quality.
- Suitable for complex data environments.
Pros
- Strong data integration capabilities.
- AI-driven data cleansing.
- Comprehensive toolset.
Cons
- Can be complex for beginners.
- Requires technical expertise for advanced features.
Requirements
- Compatible operating system (Windows, Mac, Linux).
- Sufficient RAM and CPU for large datasets.
Use Cases
- Data integration and ETL processes.
- Data quality management.
- Business intelligence and analytics.
20. TensorFlow
TensorFlow, developed by Google, is an open-source machine learning framework widely used for deep learning applications. It supports a wide range of tasks, including image recognition, natural language processing, and time-series analysis. TensorFlow’s extensive library and community support make it a powerful tool for developing and deploying AI models.
Key Features
- Extensive Library Support: Access to numerous pre-built models and libraries.
- Scalable Architecture: Supports distributed training and deployment.
- Flexibility: Adaptable to various machine learning tasks.
Strengths
- Powerful framework for deep learning and AI development.
- Strong community support and extensive resources.
- Highly flexible and scalable.
Pros
- Strong community support.
- Wide range of applications.
- Scalable and flexible.
Cons
- Steeper learning curve for beginners.
- Requires programming knowledge.
Requirements
- Python programming environment.
- Compatible operating system (Windows, Mac, Linux).
Use Cases
- Development of deep learning models.
- Image recognition and computer vision projects.
- Natural language processing and time-series analysis.
21. Microsoft Azure Machine Learning
Microsoft Azure Machine Learning is a cloud-based service that provides tools for building, training, and deploying machine learning models. It offers automated machine learning, drag-and-drop model building, and extensive integration with other Azure services. Azure Machine Learning’s scalability and comprehensive feature set make it a strong choice for enterprise AI initiatives.
Key Features
- Automated Machine Learning: Simplifies the process of building ML models.
- Drag-and-Drop Interface: Easy-to-use interface for model development.
- Extensive Integration: Works seamlessly with other Azure services.
Strengths
- Scalable cloud-based infrastructure.
- User-friendly for both beginners and experts.
- Comprehensive toolset for AI and machine learning.
Pros
- Scalable and flexible.
- Strong integration with Azure services.
- User-friendly interface.
Cons
- Can be costly for large-scale use.
- Requires internet connection.
Requirements
- Microsoft account for full functionality.
- Compatible operating system and web browser.
Use Cases
- Large-scale machine learning projects.
- Predictive analytics and forecasting.
- Integration with enterprise applications and services.
Comparing AI Data Analysis Tools – Features and Pricing
Tool | Key Features | Pros | Cons | Pricing (approx.) |
---|---|---|---|---|
Tableau | Interactive Dashboards, Data Connectivity | Excellent visualization, wide data source support | Expensive, training required | Starts at $70/user/month |
Polymer | No-Code Platform, AI-Driven Insights | No coding required, fast implementation | Limited customization, not for large datasets | Custom pricing |
Power BI | Comprehensive Analytics, Wide Data Source Support | Extensive integration, user-friendly | Complex for beginners, subscription cost | Starts at $9.99/user/month |
Akkio Inc. | Automated Machine Learning, Data Preprocessing | No coding required, quick model building | Limited customization, efficiency issues with large datasets | Custom pricing |
MonkeyLearn | Text Analysis, Customizable Models | Powerful text analytics, no coding required | Limited to text data, can be costly | Free tier available, paid plans start at $299/month |
KNIME | Visual Workflow Interface, Open-Source Platform | Free, extensive integration options | Complex for beginners, requires technical knowledge | Free |
IBM Watson Analytics | Automated Data Preparation, Natural Language Processing | Advanced AI, user-friendly | Expensive, requires internet | Custom pricing |
RapidMiner | Visual Workflow Designer, End-to-End Platform | Comprehensive toolset, strong integration | Resource-intensive, requires training | Free tier available, paid plans start at $2500/year |
Sisense | AI-Driven Analytics, Powerful Visualization | Real-time processing, strong integration | Expensive, training required | Custom pricing |
DataRobot, Inc. | Automated Machine Learning, Feature Engineering | Fast model development, user-friendly | Costly, limited customization | Custom pricing |
Qlik | Associative Engine, Advanced Analytics | Intuitive exploration, strong analytics | Complex for beginners, significant investment | Custom pricing |
AnswerRocket | Natural Language Processing, Real-Time Insights | Easy to use, real-time insights | Limited customization, expensive | Custom pricing |
Google Cloud Platform | BigQuery, AutoML, AI Platform | Powerful, scalable, wide range of tools | Complex for beginners, requires technical expertise | Pay-as-you-go |
PyTorch | Dynamic Computational Graph, Extensive Library Support | Strong community, flexible | Steep learning curve, requires programming | Free |
ChatGPT | Natural Language Understanding, Conversational AI | Easy to use, versatile | Limited to conversational use, requires internet | Free tier available, custom pricing for advanced use |
Databricks | Unified Analytics Platform, Apache Spark Integration | Scalable, strong collaboration | Expensive, requires technical expertise | Custom pricing |
Jupyter AI | Interactive Notebooks, AI-Driven Features | Interactive, strong library support | Requires programming, resource-intensive | Free |
Luzmo | Real-Time Insights, Data Integration | Real-time analytics, user-friendly | Costly for larger datasets, training required | Custom pricing |
Talend | Data Integration, AI-Driven Data Cleansing | Strong integration, comprehensive toolset | Complex for beginners, requires technical expertise | Free tier available, paid plans start at $1200/year |
TensorFlow | Extensive Library Support, Scalable Architecture | Strong community, scalable | Steep learning curve, requires programming | Free |
Microsoft Azure Machine Learning | Automated Machine Learning, Drag-and-Drop Interface | Scalable, strong Azure integration | Costly, requires internet | Pay-as-you-go |
Choosing the best AI tools for data analysis depends on your specific needs, technical expertise, and budget. The above comparison should help you identify the best tool suited to your requirements.